As someone who identifies with the LGBT+ community in Vietnam, Võ Tuấn Sơn is particularly interested in gender-related issues. Growing up in the age of social media and digital technology, he also has a fascination with how the tech world interacts with gender in human society. These two topics were combined in his Digital Anthropology master’s program at the University College London in the UK.
Back in Vietnam, after completing his master’s degree, Võ Tuấn Sơn became a policy analyst specializing in digital transformation at the World Bank. While providing expert advice to public institutions, he noticed the importance of considering the social aspects when applying artificial intelligence tools to everyday life. Here, Võ Tuấn Sơn talks about how AI inherits human biases.
The manifestation of biases
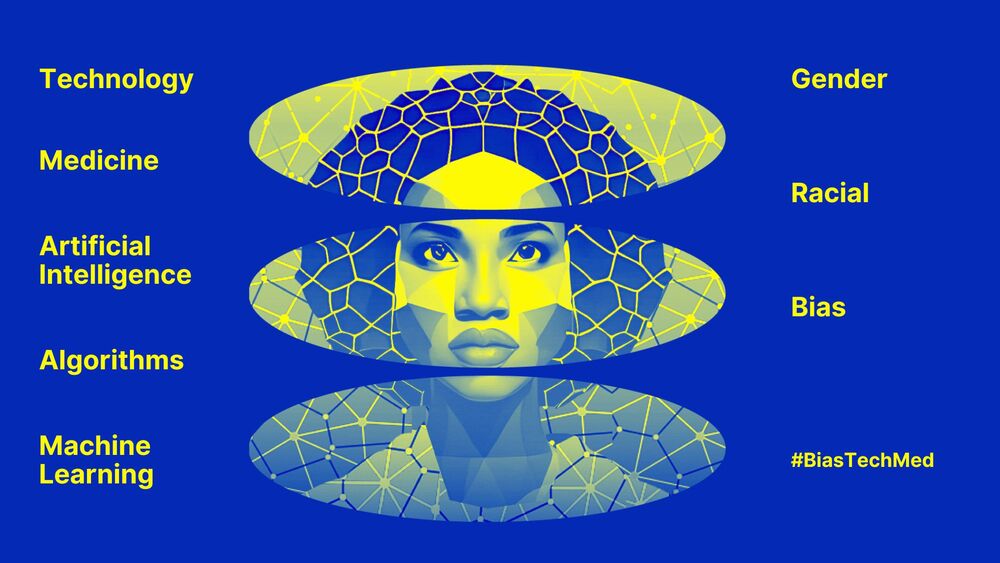
In recent years, Amazon receives over a million job applications annually. About a decade ago, they adopted AI technology to speed up their hiring process. However, this breakthrough hiring tool didn’t “favor” women for positions like software engineers or programmers. In other words, Amazon’s AI exhibited gender biases when it came to hiring in the information technology field.
AI errors are quite common worldwide. Google’s image processing application faced criticism for racial discrimination when it identified a black woman as a “gorilla.” Tay - a chatbot using Microsoft’s AI technology on Twitter - was shut down within less than 24 hours of its launch due to its gender and racially discriminatory remarks. Genderify, a platform that analyzes names and email addresses to determine gender, also faced criticism for its biases. For example, when inputting the name “Meghan Smith,” the result showed 39.6% male and 60.4% female, but when adding “Dr.” (Doctor) to become “Dr. Meghan Smith,” the result shifted to 75.9% male and 24.1% female.
AI technology is not purely about machines that can evaluate and process information “objectively” better than humans. AI technology can inherit human biases and amplify them in the digital environment as a product of human creation.
Why can AI have biases?
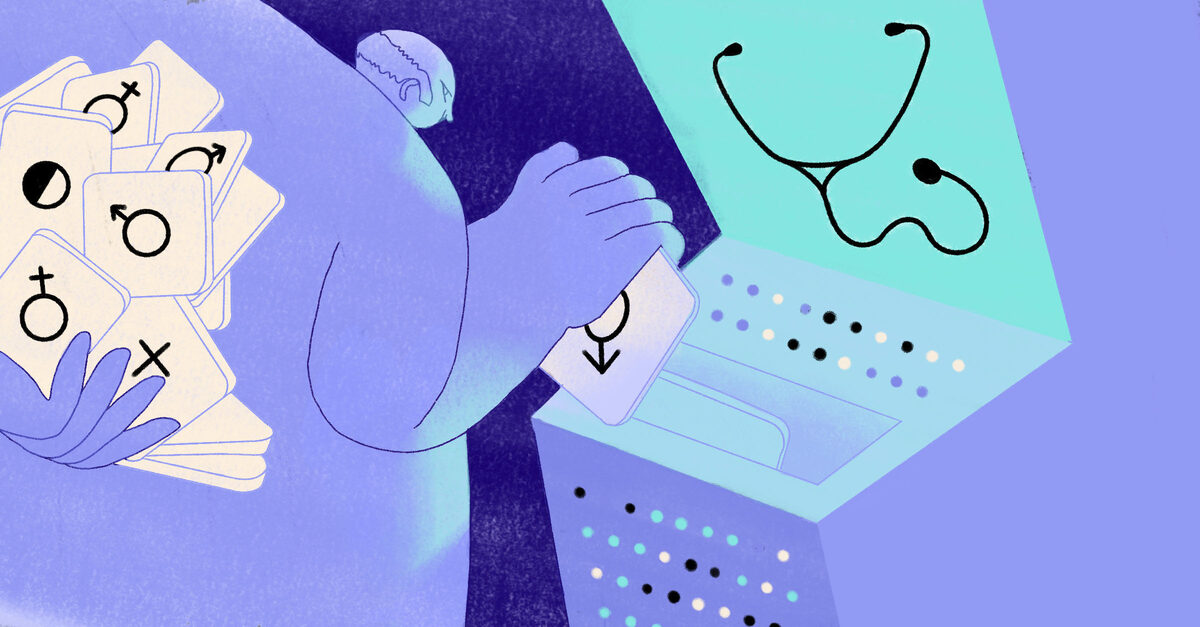
First, we need to understand what biases are. Biases are viewpoints reinforced by human preconceptions, supporting or opposing individuals, groups, or beliefs. Gender biases are reinforced by human preconceptions and beliefs about gender formed from specific social and historical contexts.
Common gender biases include associating professions like “nurse," “housekeeper,” “secretary,” and “homemaker” with women, while professions like “doctor,” “soldier,” “firefighter,” and “programmer” are associated with men. These professions are gendered due to beliefs and views about the inherent nature of femininity (e.g., meticulousness, gentleness) and masculinity (e.g., decisiveness, strength). Over time, these biases become fixed, reiterated through media, education, policies, and more. To answer why AI can have biases similar to humans, we need to examine the assumption that machines, data, and algorithms are unbiased or have the capability to produce neutral outcomes. In other words, data, algorithms, and machines can produce an “objective truth."
We tend to think that data can be objectively measured because: It can be quantified and calculated with numbers; it exists in a “raw” state - unprocessed and uninterpreted; it is collected by careful, objective scientists without biases. Similarly, an algorithm is considered to reflect comprehensive truth because it processes automatically, without human intervention, when feeding data into the machine learning model and is too complex for humans to comprehend.
However, in reality, data is social and incredibly dynamic, not just “raw materials” that can be quantified. Data collected in any way reflect the ideologies, biases, and agendas of those who gather it. When answering survey questions, our information is categorized into theoretical frameworks or assigned to human-created notions, such as “introverted” or “extroverted,” “high-achieving student” or “average student,” customer segmentation classifications, or “male,” “female,” and “non-binary.”
Once fed into machine learning models/algorithms, this data - instructions and commands programmed in computer language - is processed to produce specific outcomes (e.g., algorithms suggesting videos or movies on YouTube or Netflix or songs on Spotify). Hence, every operation involving data and algorithms involves human presence.
Their creators’ biases influence the data, algorithms, and machines they build. These biases often relate to human identity characteristics like race, gender, moral values, etc. In some cases, AI technology amplifies gender biases by reinforcing them and presenting the outputs as objective and natural. We need to ask: Who collects the data, does the programming, selects the machine learning models, etc.? Do they have gender biases?
Where do biases exist in the AI operation process?
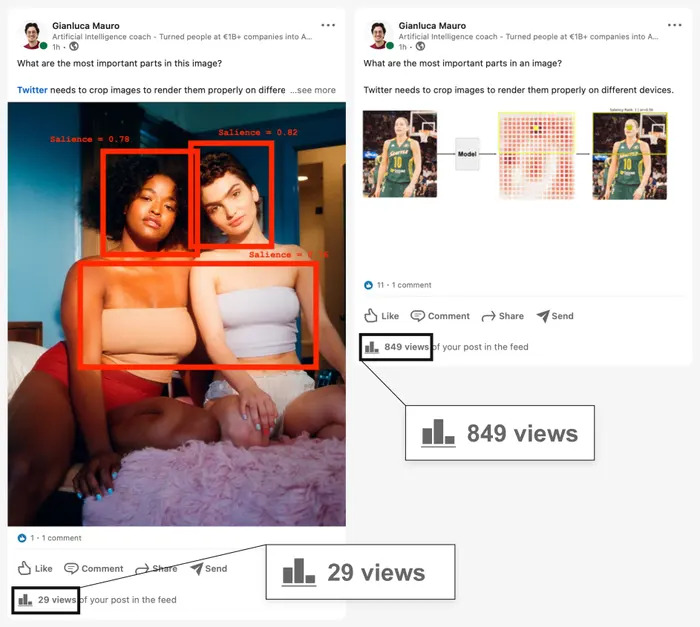
We also need to understand how AI technology works to identify where biases exist in its operational process. AI operates based on data, algorithms/machine learning models, enabling computers to automatically process intelligent behaviors similar to humans to solve specific problems.
There are various ways to classify AI technology based on its level of development or function. Regarding classification, we can refer to branches of AI development such as Natural Language Processing (NLP), which processes text to help computers understand written or spoken language like humans: virtual assistants (Siri), translation (Google), filling in words in emails, Facebook suggestions, or branches in Computer Vision, which processes images to help computers recognize and analyze objects in images and videos like human eyes: facial recognition, gesture recognition on phones, TikTok filters, etc.
There are several crucial steps in the AI system training process: Identifying the problem to be solved (e.g., predicting the cancellation rate of Adobe or Microsoft product subscriptions); Data preparation: collecting, processing, “cleaning,” and labeling data; Choosing the model/algorithm for machine learning; Testing and running data through the machine; Machine learning from the data; Evaluating errors and making adjustments; Implementing into applications.
Gender biases can appear in different steps, such as model/algorithm selection, data preparation, or real-world application. The expression of biases becomes evident in the “Implementation" step.
For example, when you type “secretary” or “nurse” on Google, there’s a predominance of female images displayed. In contrast, searching for keywords like “businessperson” or “pilot” mostly shows male images. The image data for machine learning lacks gender representation. These images are distributed widely across various cultural backgrounds and geographical regions, instantly appearing as if an obvious truth without time for consideration. These results reinforce gender biases related to human professions.
Returning to Amazon’s AI technology used for candidate selection. The task is to present 100 CVs for the machine to choose the top 5 candidates. The data is scanned and analyzed for common patterns among the CVs submitted to the company over the past 10 years. The 5 selected candidates are all men, partly because men dominate the field of information technology. However, if we only focus on the results, we are overlooking the social context where women face discrimination and more challenges than men when building careers in general and working in the field of information technology specifically.
In these examples, the “data preparation” step is crucial in shaping the manifestations of gender biases. Google’s image processing technology mostly shows female images labeled as ’ secretaries.’ Most of the analyzed CVs at Amazon labeled as “meeting criteria” are from male candidates - a group less affected because of gaps in their resumes due to maternity leave, family caregiving responsibilities, or judgments like “near-sighted programming, looks ugly” like female candidates. UNESCO has reported on gender biases in using audio data to train voice assistants like Siri and Alexa. These voice assistants used to default to a female voice due to traits like “always helpful” and “compliant,” often associated with femininity, closer to the image of a “secretary” or “housekeeper.”
All these issues stem from skewed data sets used for training AI technology, where the samples are not diverse or balanced enough. Lack of diversity in demographic factors during the “data preparation” step is also a significant issue leading to manifestations of gender biases.
Can technology be unbiased?
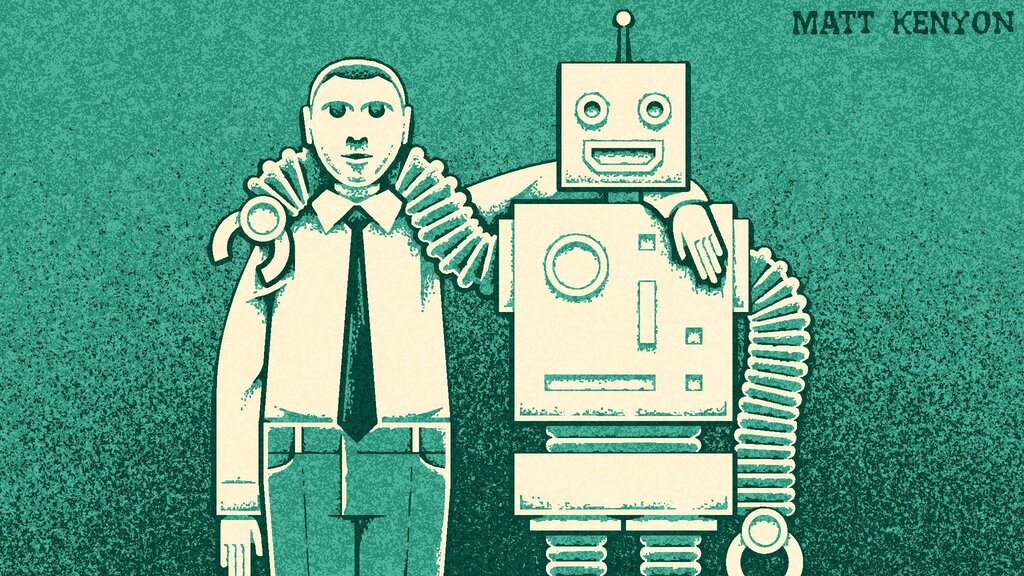
If unbiased means AI technology can be neutral and objective, then no. For AI technology to function, it requires a dataset. Having a dataset means having human input. Human input entails the potential for biases and prejudices. Every framework, labeling approach, or general knowledge is linked to humans and specific contexts. Hence, that knowledge won’t be objective.
However, the potential risks of AI technology are real. Around the world, legislators, businesses, and individuals are debating and devising solutions to reduce biases and manage risks from AI technology.
On a personal level, we can ponder questions like, “What are our gender biases? Are our jobs reinforcing gender biases? How can we reduce biases?” Considering culture and specific contexts is also useful for making AI technology and biases more relatable to us.
Some common gender patterns and existing beliefs in Vietnam include the notion of male superiority, the pillar of the family, and the expectation that women must embody the Four Virtues - housekeeping skills, beauty, appropriate speech, and moral conduct. These beliefs are often reinforced by commercial slogans like “chuẩn men” (real man) or “là con gái phải xinh” (girls must be beautiful).
While there might be some similarities, Vietnamese gender patterns and beliefs, such as the idea of breadwinners and caregivers, may differ from those in other countries and regions. Vietnamese beliefs may also include other notions, like women in the family always being “the keyholder.”
For those working in technology-related fields, these questions and reflections become essential.
Additionally, data feminists at MIT have suggested principles for approaching data, such as analyzing power relations in society, reflecting on binary structures and hierarchical systems, ensuring participant diversity for projects involving data or AI technology, and describing the context of data practices. These principles help us not rely on the “objectivity” of data and acknowledge its specific biases and social effects. Thus, we don’t take AI technology’s results and solutions for granted without questioning them.
Legal Governance of AI
In 2021, UNESCO adopted the Recommendation on the Ethics of Artificial Intelligence, based on four core values “Respect, protection and promotion of human rights and fundamental freedoms and human dignity,” “Living in peaceful, just and interconnected societies," “Environment and ecosystem flourishing,” and “Ensuring diversity and inclusiveness.”
These recommendations focus on specific themes such as “Right to Privacy and Data Protection,” “Responsibility and accountability,” and ensuring the “Transparency and explainability” of AI technology. The recommendations also address promoting gender equality in AI governance, emphasizing principles of “fairness and non-discrimination” and suggesting policies and investment for women and girls’ education and work in STEM fields.
Countries like Japan, South Korea, Singapore, Spain, the United Kingdom, and the United States have enacted various laws and policies to govern AI technology. In Vietnam, steps have been taken toward AI governance, such as the issuance of Decision No. 127/2021/QD-TTg on the “National Strategy on Research, Development, and Application of Artificial Intelligence until 2030.” Moreover, after several delays, the Vietnamese government adopted Decree No. 13/2023/ND-CP on “Personal Data Protection” in April 2023, drawing inspiration from the EU’s General Data Protection Regulation (GDPR). Vietnam is also learning from UNESCO’s recommendations on AI ethics and other guiding documents to establish a legal framework for AI governance.
However, a 2022 study on AI development in Southeast Asia revealed that Vietnam still faces gender imbalances in STEM fields (at the university level, females represent 36.5% compared to males at 63.5%), leading to potential biases, marginalization, and discrimination against women in the AI industry. Furthermore, the study indicated that awareness and language concerning AI ethics, including the impact of AI technology on gender inequality, remain insufficiently widespread in daily life.
AI, in particular, and technology, in general, have introduced a new dimension to the fight against gender inequality. While technology holds great potential, it is not a one-size-fits-all solution to this issue. Addressing these challenges requires a systemic approach and engagement in various aspects such as law, economy, education, etc., as these dimensions are interconnected with technology and gender bias.